// Sample DataFrames
val tokens = Seq(
(0, "playing"),
(1, "with"),
(2, "InMemoryTableScanExec")
).toDF("id", "token")
val ids = spark.range(10)
// Cache DataFrames
tokens.cache
ids.cache
val q = tokens.join(ids, Seq("id"), "outer")
scala> q.explain
== Physical Plan ==
*Project [coalesce(cast(id#5 as bigint), id#10L) AS id#33L, token#6]
+- SortMergeJoin [cast(id#5 as bigint)], [id#10L], FullOuter
:- *Sort [cast(id#5 as bigint) ASC NULLS FIRST], false, 0
: +- Exchange hashpartitioning(cast(id#5 as bigint), 200)
: +- InMemoryTableScan [id#5, token#6]
: +- InMemoryRelation [id#5, token#6], true, 10000, StorageLevel(disk, memory, deserialized, 1 replicas)
: +- LocalTableScan [id#5, token#6]
+- *Sort [id#10L ASC NULLS FIRST], false, 0
+- Exchange hashpartitioning(id#10L, 200)
+- InMemoryTableScan [id#10L]
+- InMemoryRelation [id#10L], true, 10000, StorageLevel(disk, memory, deserialized, 1 replicas)
+- *Range (0, 10, step=1, splits=8)
InMemoryTableScanExec Physical Operator
InMemoryTableScanExec
is a leaf physical operator that…FIXME
InMemoryTableScanExec
is created exclusively when InMemoryScans execution planning strategy finds InMemoryRelation
logical operators.
Name | Description |
---|---|
Number of output rows |
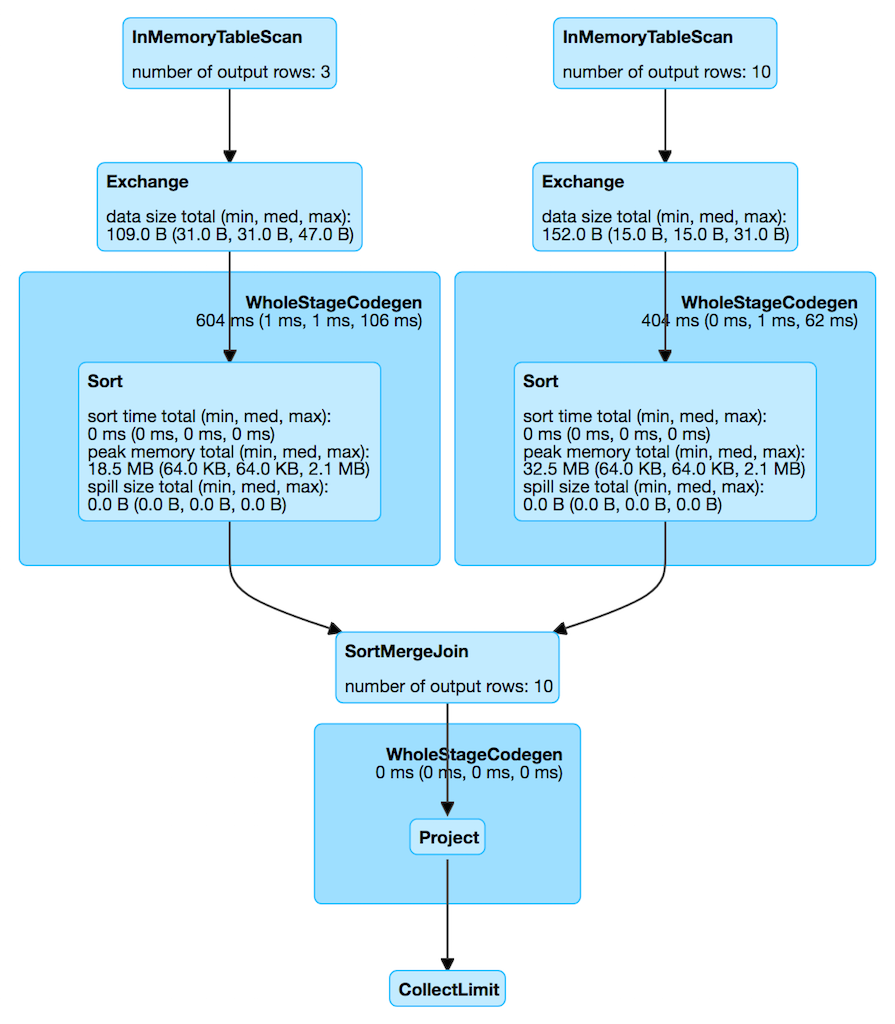
Figure 1. InMemoryTableScanExec in web UI (Details for Query)
InMemoryTableScanExec
uses spark.sql.inMemoryTableScanStatistics.enable
flag (default: disabled) to enable accumulators (that appears exclusively for testing purposes).
Creating InMemoryTableScanExec Instance
InMemoryTableScanExec
takes the following when created:
-
Attribute expressions
-
Predicate expressions
-
InMemoryRelation logical operator