sc.newAPIHadoopFile("filepath1, filepath2", classOf[NewTextInputFormat], classOf[LongWritable], classOf[Text])
Using Input and Output (I/O)
Caution
|
FIXME What are the differences between textFile and the rest methods in SparkContext like newAPIHadoopRDD , newAPIHadoopFile , hadoopFile , hadoopRDD ?
|
Spark is like Hadoop - uses Hadoop, in fact - for performing actions like outputting data to HDFS. You’ll know what I mean the first time you try to save "all-the-data.csv" and are surprised to find a directory named all-the-data.csv/ containing a 0 byte _SUCCESS file and then several part-0000n files for each partition that took part in the job.
The read operation is lazy - it is a transformation.
Methods:
-
SparkContext.textFile(path: String, minPartitions: Int = defaultMinPartitions): RDD[String] reads a text data from a file from a remote HDFS, a local file system (available on all nodes), or any Hadoop-supported file system URI (e.g. sources in HBase or S3) at
path
, and automatically distributes the data across a Spark cluster as an RDD of Strings.-
Uses Hadoop’s org.apache.hadoop.mapred.InputFormat interface and file-based org.apache.hadoop.mapred.FileInputFormat class to read.
-
Uses the global Hadoop’s
Configuration
with allspark.hadoop.xxx=yyy
properties mapped toxxx=yyy
in the configuration. -
io.file.buffer.size
is the value ofspark.buffer.size
(default:65536
). -
Returns HadoopRDD
-
When using
textFile
to read an HDFS folder with multiple files inside, the number of partitions are equal to the number of HDFS blocks.
-
-
What does
sc.binaryFiles
?
URLs supported:
-
s3://…
ors3n://…
-
hdfs://…
-
file://…;
The general rule seems to be to use HDFS to read files multiple times with S3 as a storage for a one-time access.
Creating RDDs from Input
Saving RDDs to files - saveAs* actions
An RDD can be saved to a file using the following actions:
-
saveAsTextFile
-
saveAsObjectFile
-
saveAsSequenceFile
-
saveAsHadoopFile
Since an RDD is actually a set of partitions that make for it, saving an RDD to a file saves the content of each partition to a file (per partition).
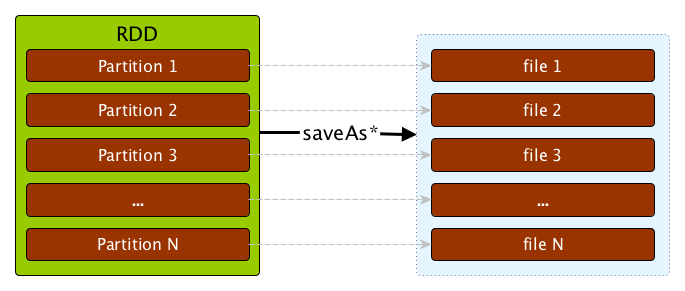
If you want to reduce the number of files, you will need to repartition the RDD you are saving to the number of files you want, say 1.
scala> sc.parallelize(0 to 10, 4).saveAsTextFile("numbers") (1)
...
INFO FileOutputCommitter: Saved output of task 'attempt_201511050904_0000_m_000001_1' to file:/Users/jacek/dev/oss/spark/numbers/_temporary/0/task_201511050904_0000_m_000001
INFO FileOutputCommitter: Saved output of task 'attempt_201511050904_0000_m_000002_2' to file:/Users/jacek/dev/oss/spark/numbers/_temporary/0/task_201511050904_0000_m_000002
INFO FileOutputCommitter: Saved output of task 'attempt_201511050904_0000_m_000000_0' to file:/Users/jacek/dev/oss/spark/numbers/_temporary/0/task_201511050904_0000_m_000000
INFO FileOutputCommitter: Saved output of task 'attempt_201511050904_0000_m_000003_3' to file:/Users/jacek/dev/oss/spark/numbers/_temporary/0/task_201511050904_0000_m_000003
...
scala> sc.parallelize(0 to 10, 4).repartition(1).saveAsTextFile("numbers1") (2)
...
INFO FileOutputCommitter: Saved output of task 'attempt_201511050907_0002_m_000000_8' to file:/Users/jacek/dev/oss/spark/numbers1/_temporary/0/task_201511050907_0002_m_000000
-
parallelize
uses4
to denote the number of partitions so there are going to be 4 files saved. -
repartition(1)
to reduce the number of the files saved to 1.
S3
s3://…
or s3n://…
URL are supported.
Upon executing sc.textFile
, it checks for AWS_ACCESS_KEY_ID
and AWS_SECRET_ACCESS_KEY
. They both have to be set to have the keys fs.s3.awsAccessKeyId
, fs.s3n.awsAccessKeyId
, fs.s3.awsSecretAccessKey
, and fs.s3n.awsSecretAccessKey
set up (in the Hadoop configuration).
textFile reads compressed files
scala> val f = sc.textFile("f.txt.gz")
f: org.apache.spark.rdd.RDD[String] = MapPartitionsRDD[5] at textFile at <console>:24
scala> f.foreach(println)
...
15/09/13 19:06:52 INFO HadoopRDD: Input split: file:/Users/jacek/dev/oss/spark/f.txt.gz:0+38
15/09/13 19:06:52 INFO CodecPool: Got brand-new decompressor [.gz]
Ala ma kota
Reading Sequence Files
-
sc.sequenceFile
-
if the directory contains multiple
SequenceFiles
all of them will be added to RDD
-
-
SequenceFile RDD
Changing log levels
Create conf/log4j.properties
out of the Spark template:
cp conf/log4j.properties.template conf/log4j.properties
Edit conf/log4j.properties
so the line log4j.rootCategory
uses appropriate log level, e.g.
log4j.rootCategory=ERROR, console
If you want to do it from the code instead, do as follows:
import org.apache.log4j.Logger
import org.apache.log4j.Level
Logger.getLogger("org").setLevel(Level.OFF)
Logger.getLogger("akka").setLevel(Level.OFF)
FIXME
Describe the other computing models using Spark SQL, MLlib, Spark Streaming, and GraphX.
$ ./bin/spark-shell
...
Spark context available as sc.
...
SQL context available as spark.
Welcome to
____ __
/ __/__ ___ _____/ /__
_\ \/ _ \/ _ `/ __/ '_/
/___/ .__/\_,_/_/ /_/\_\ version 1.5.0-SNAPSHOT
/_/
Using Scala version 2.11.7 (Java HotSpot(TM) 64-Bit Server VM, Java 1.8.0_60)
Type in expressions to have them evaluated.
Type :help for more information.
scala> sc.addFile("/Users/jacek/dev/sandbox/hello.json")
scala> import org.apache.spark.SparkFiles
import org.apache.spark.SparkFiles
scala> SparkFiles.get("/Users/jacek/dev/sandbox/hello.json")
Caution
|
Review the classes in the following stacktrace. |
scala> sc.textFile("http://japila.pl").foreach(println)
java.io.IOException: No FileSystem for scheme: http
at org.apache.hadoop.fs.FileSystem.getFileSystemClass(FileSystem.java:2644)
at org.apache.hadoop.fs.FileSystem.createFileSystem(FileSystem.java:2651)
at org.apache.hadoop.fs.FileSystem.access$200(FileSystem.java:92)
at org.apache.hadoop.fs.FileSystem$Cache.getInternal(FileSystem.java:2687)
at org.apache.hadoop.fs.FileSystem$Cache.get(FileSystem.java:2669)
at org.apache.hadoop.fs.FileSystem.get(FileSystem.java:371)
at org.apache.hadoop.fs.Path.getFileSystem(Path.java:295)
at org.apache.hadoop.mapred.FileInputFormat.singleThreadedListStatus(FileInputFormat.java:258)
at org.apache.hadoop.mapred.FileInputFormat.listStatus(FileInputFormat.java:229)
at org.apache.hadoop.mapred.FileInputFormat.getSplits(FileInputFormat.java:315)
at org.apache.spark.rdd.HadoopRDD.getPartitions(HadoopRDD.scala:207)
at org.apache.spark.rdd.RDD$$anonfun$partitions$2.apply(RDD.scala:239)
at org.apache.spark.rdd.RDD$$anonfun$partitions$2.apply(RDD.scala:237)
at scala.Option.getOrElse(Option.scala:121)
at org.apache.spark.rdd.RDD.partitions(RDD.scala:237)
at org.apache.spark.rdd.MapPartitionsRDD.getPartitions(MapPartitionsRDD.scala:35)
at org.apache.spark.rdd.RDD$$anonfun$partitions$2.apply(RDD.scala:239)
at org.apache.spark.rdd.RDD$$anonfun$partitions$2.apply(RDD.scala:237)
at scala.Option.getOrElse(Option.scala:121)
at org.apache.spark.rdd.RDD.partitions(RDD.scala:237)
...